2022 is the year of text to image generators so far. With a crazy hunt for new tools starting in summer. Following some GANs of 2021, such as VQGAN and others, I mentioned in my article “Speaking computer language – welcome AI Art", the hunt for the next generation of text-to-image generation started in April when OpenAI introduced the magic of DALL·E 2.
During the year I shared articles around the topic of AI tools for image generation and if you had asked me in January, I wouldn't have expected such a development. I tested midjourney and DALL·E 2 and shared my thoughts on different ways to utilize AI tools. There's also Google launching more and more new tools on the market, one of the latest is DreamBooth, something that could be added to the workflows of the broadly available text-to-image tools, but that's another story.
While finishing my review on midjourney and DALL·E 2, I noticed the official launch of Stable Diffusion. Then I struggled, as Stable Diffusion is different from all the other tools before: it’s Open Source. Being Open Source, it is a creativity machine on its own, as it is possible to connect it to other tools as well. My core question was… where to start to share my thoughts? Explaining prompt guides as for midjourney or DALL·E 2?
In my following article I wanted to give a broad overview of the current status quo as of September 2022. Ways to use Stable Diffusion and share possibilities of open source software happening currently.
What a good artist understands is that nothing comes from nowhere. All creative work builds on what came before. Nothing is completely original.
from Steal Like an Artist: 10 Things Nobody Told You About Being Creative.
Stable Diffusion – what is it?
Stable Diffusion is a model that can create digital images from text or use text prompts to guide image-to-image translations. It will be released on August 22, 2022.Looking at the research paper you will notice that some names you might have come across in former publications on GANs will show up again, so all people being mentioned in the research paper of VQGAN are mentioned: Patrick Esser, Dominik Lorenz and Robin Rombach.
Understanding the heritage can help comprehend the rapid development of VQGAN in just one year. The progress has been extraordinary.
Currently, all main competitors (DALL·E 2, midjourney and Stable diffusion) rely on the principle of diffusion to create their images, so-called latent diffusion models. If you want to get a deeper understanding, I recommend a good written article from Louis Bouchard or watch his video:
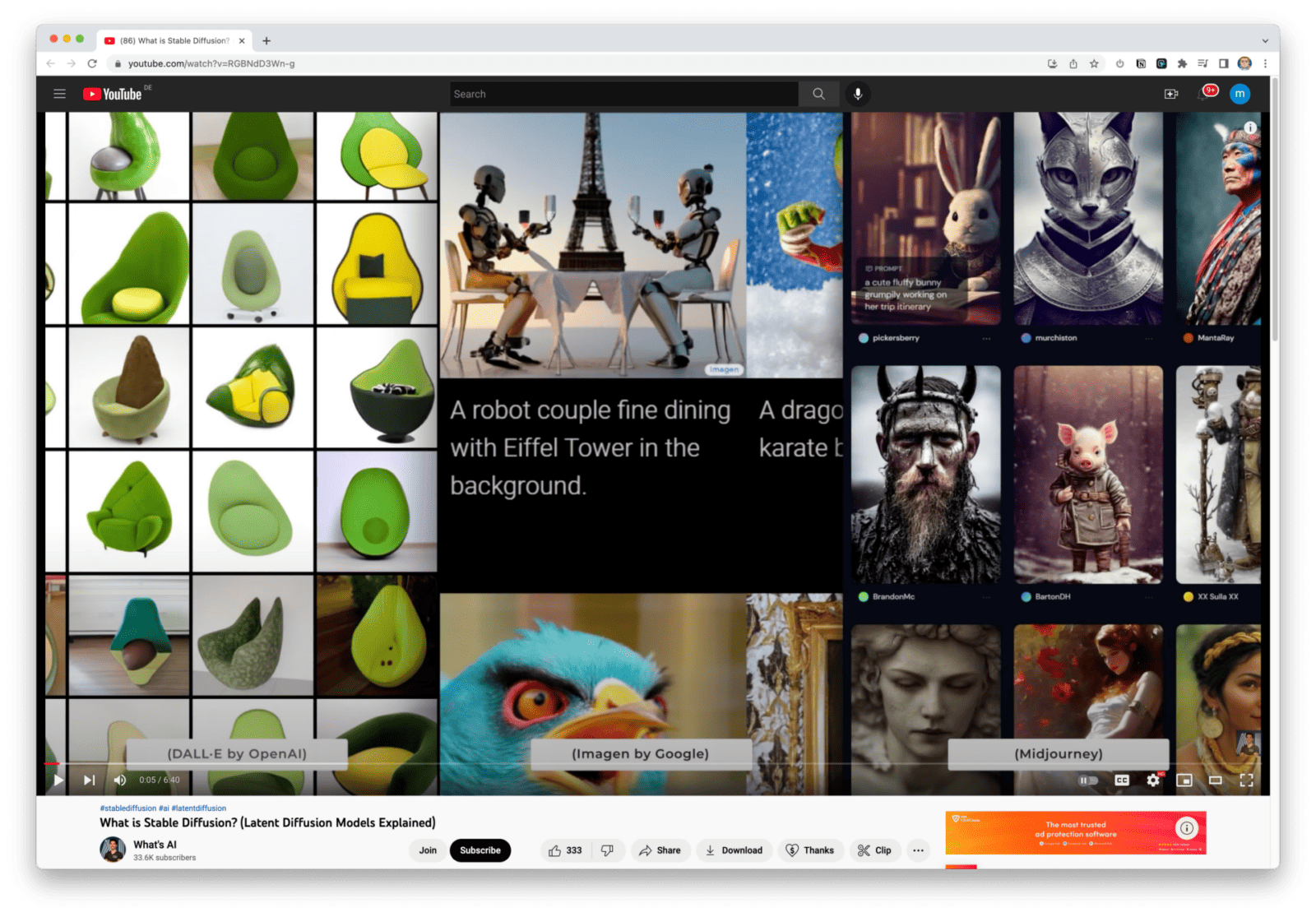
Looking at results with VQGAN and Clip, see my older article and results from one year later, give you an idea about the speed of development. We face many competitors and an impressive research speed. I assume the next challenge is render speed while generating the images and optimizing the diffusion models. In the meantime, the world is allowed to unleash its full potential by connecting more libraries together to Stable Diffusion.
I really recommend trying some of the text-prompts yourself. Generating images on your computer can be difficult. The process involves using language prompts and the speed of image generation tells you how complex it is. On my MacBook (with m1) it’s running ok, but I am not sure on which PC builds you could run it.
Playing with the software, you'll observe cases the current implementations struggle with - one of the harder ones is stigmatizing the world around us. No wonder if the models are trained by common images of the web. The most common pictures on the web are pictures more beautiful than reality.
Watch "Stable Diffusion - What, Why, How?" by Edan Meyer, a one-hour instructional video on creating diffusion videos. It's presented greatly and a great resource to get started with Stable Diffusion.
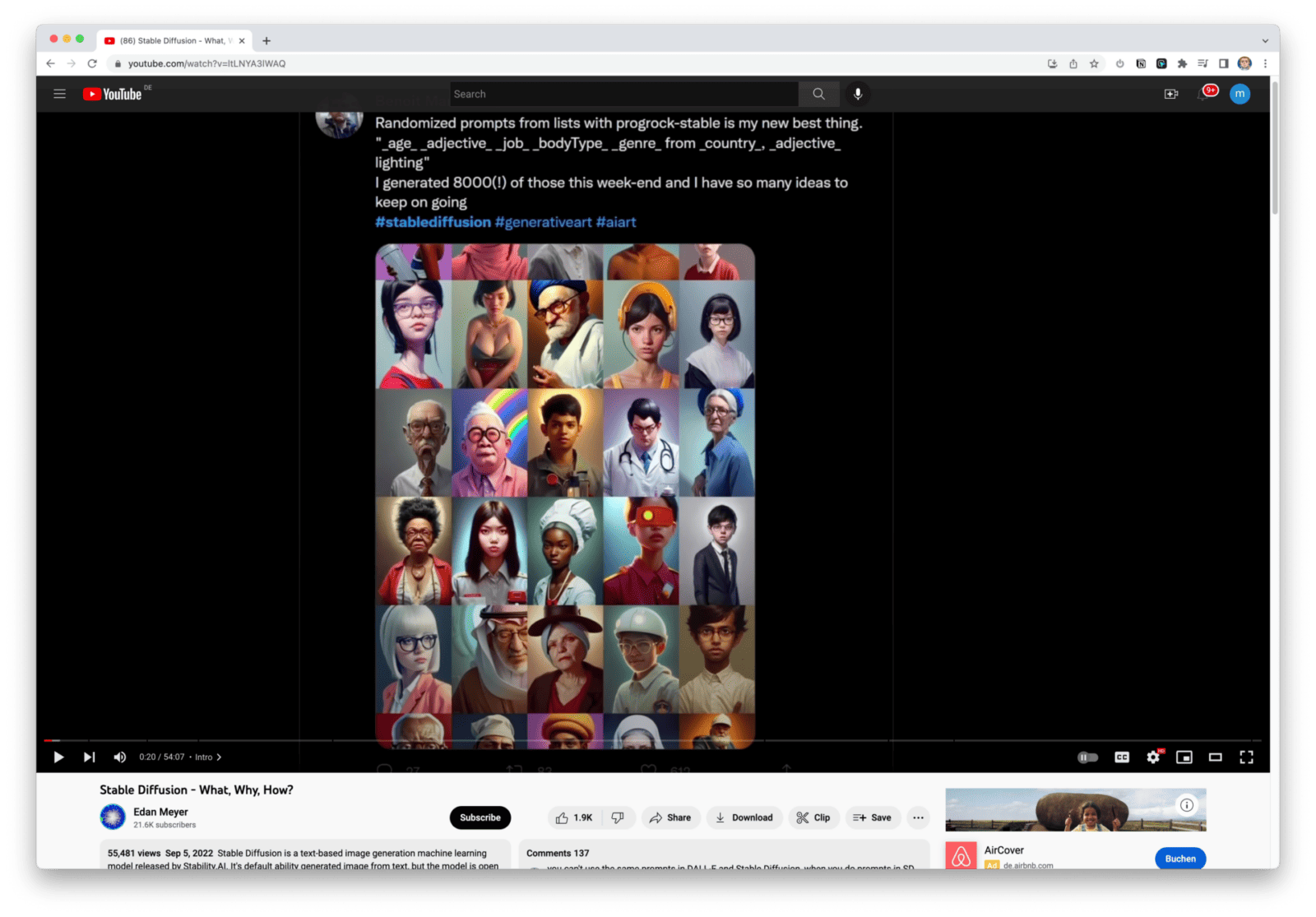
If you want a shorter version of Stable Diffusion, look at the 2-minute paper.
Stable Diffusion – Quickstart via dreamstudio, collab, huggingface
If you had a look at midjourney or DALL·E 2 before, you experienced simple text-to-image prompts to create your artworks. Being Open Source opens a variety of inputs for Stable Diffusion. I try to give you an overview (with a raise in complexity and flexibility).
Dreamstudio.ai
Dreamstudio is the easy access to Stable Diffusion and the answer to popular midjourney or DALL·E 2. To get started, you need to login. Also, the prompt guide is only available when logged in.
The main difference to midjourney (using discord) and DALL·E 2 (basically relying on the text prompt) is the interface you get provided. Besides a text prompt you also get some rulers to modify the settings. Though the wording being used is still geeky, it is progress to the simple text inputs from the other main rivals. By the way, there also used to be an official discord bot, but they shut it down.
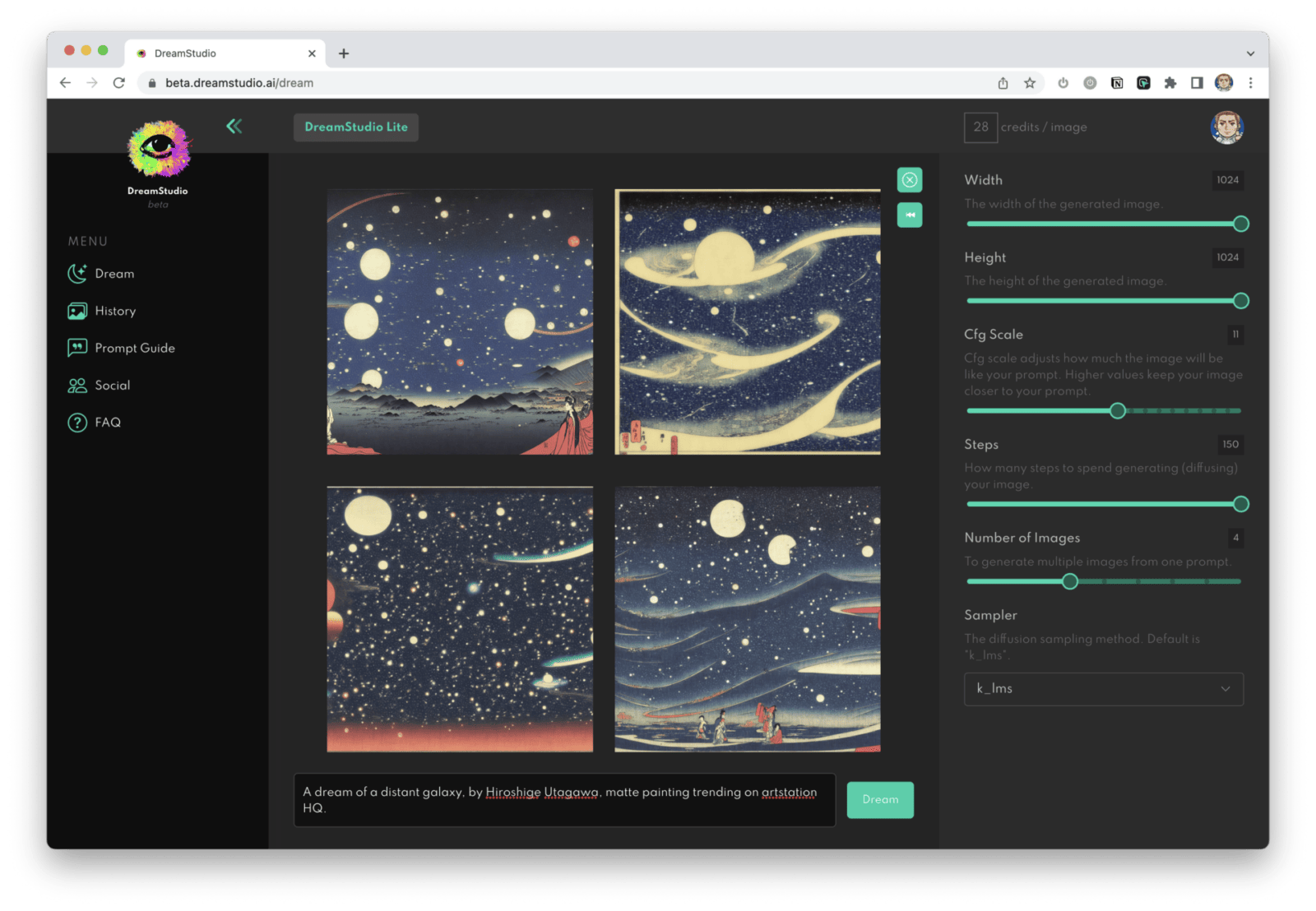
The payment model for dreamstudio feels more complicated than for midjourney or DALL·E 2. You also get free credits to get started but the amount of cost varies with your settings; here’s their explanation:
At default settings, it’s one credit per image. Depending on the image resolution & step count you choose, this can go as low as 0.2 credits per image or as high as 28.2 credits per image. Here is a detailed chart for explanation:
Steps | 512x512 | 512x768 | 512x1024 | 768x768 | 768x1024 | 1024x1024 |
---|---|---|---|---|---|---|
10 | 0.2 | 0.5 | 0.8 | 0.9 | 1.3 | 1.9 |
25 | 0.5 | 1.2 | 1.9 | 2.3 | 3.3 | 4.7 |
50 | 1.0 | 2.4 | 3.8 | 4.6 | 6.6 | 9.4 |
75 | 1.5 | 3.6 | 5.7 | 6.9 | 9.9 | 14.1 |
100 | 2.0 | 4.8 | 7.6 | 9.2 | 13.2 | 18.8 |
150 | 3.0 | 7.2 | 11.4 | 13.8 | 19.8 | 28.2 |
If you need some extra guidance on the photo editor, watch the video from their FAQs: Getting Started With DreamStudio Website Beta, Part Five: Inpainting/Outpainting.
In the FAQs you will find a lot of other helpful sources to get started.
All in all, it’s cool to have a maintained/managed tool showing the potential of Stable Diffusion and easily experiment. If you like what you see, you could continue to pay or switch to Open Source.
As you have seen, the tool also has some limitations at the moment: the size of the images is limited to 1024x1024 (midjourney allows 2048x2048). The size is directly connected to the render speed.
But no worries about the size: one of the biggest sins in photo editing is also a relic of the past. Upscaling pictures is now possible with the help of other AI image upscaling tools. There are so many tools nowadays, maybe you find some inspiration in my other article “How AI Assistants Can Help You with Text and Art”.
You can also look for GIT repositories to size the images. One I could recommend is this txt2imghd. There's also a version on replicate.com.
Stable Diffusion on Huggingface.co
You must have come across hugging face if you got into text to image before. I shared some stuff from hugging face before, such as Dalle-mini, a free Dalle alternative from earlier this year. I really recommend anyone being interested in the topic of AI and machine learning, to visit hugging face every now and then. Under “spaces” you will find apps you could directly test as web-apps. And you notice (sorted by likes) that there’s also an app for Stable Diffusion.
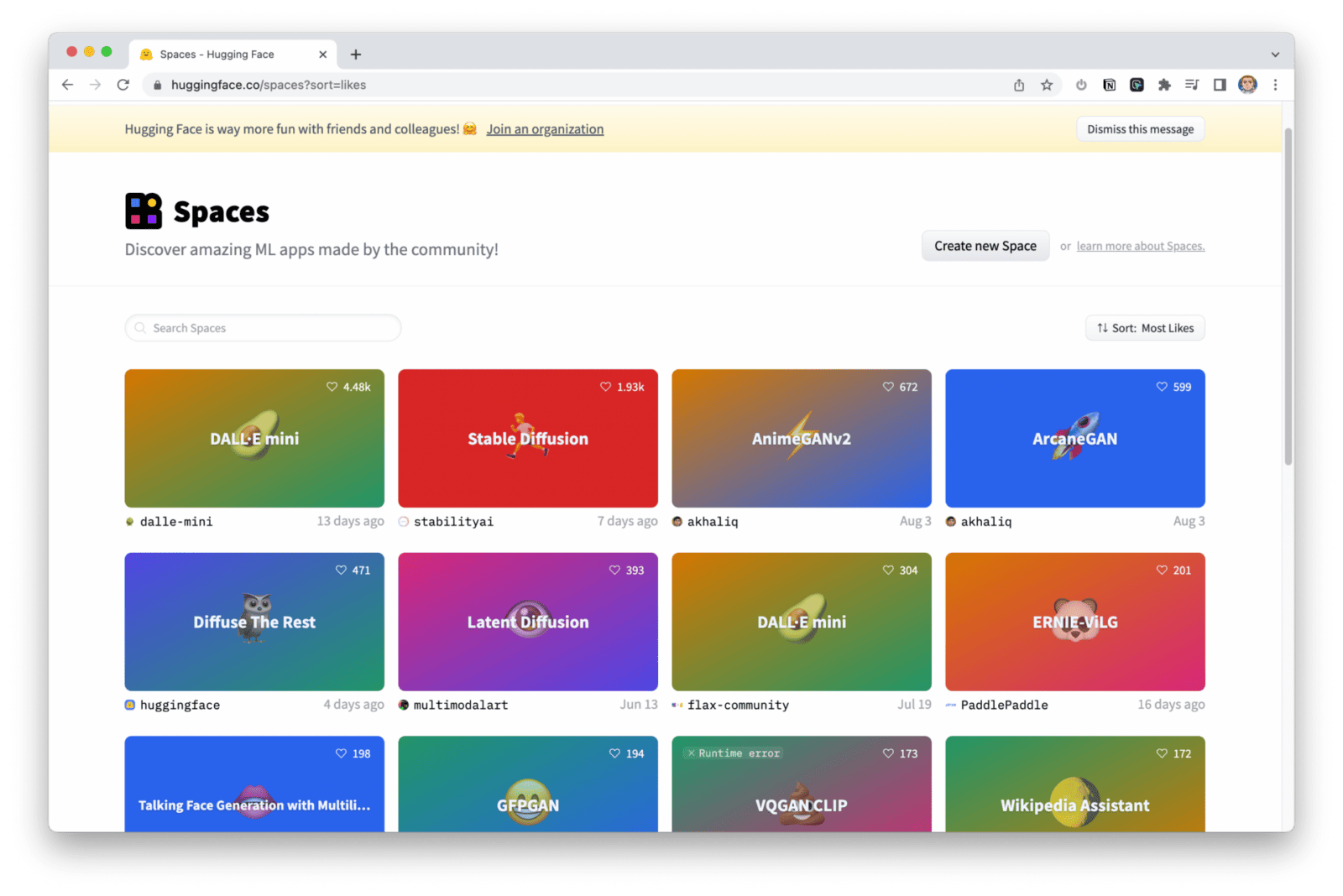
In fact, there are more apps using Stable Diffusion to transform images. The one on the left is the simple text to image prompt and the right one could be used for modifying pictures as well. Feel free to try one of the two here:
Here are some of the outputs being generated in the tool:
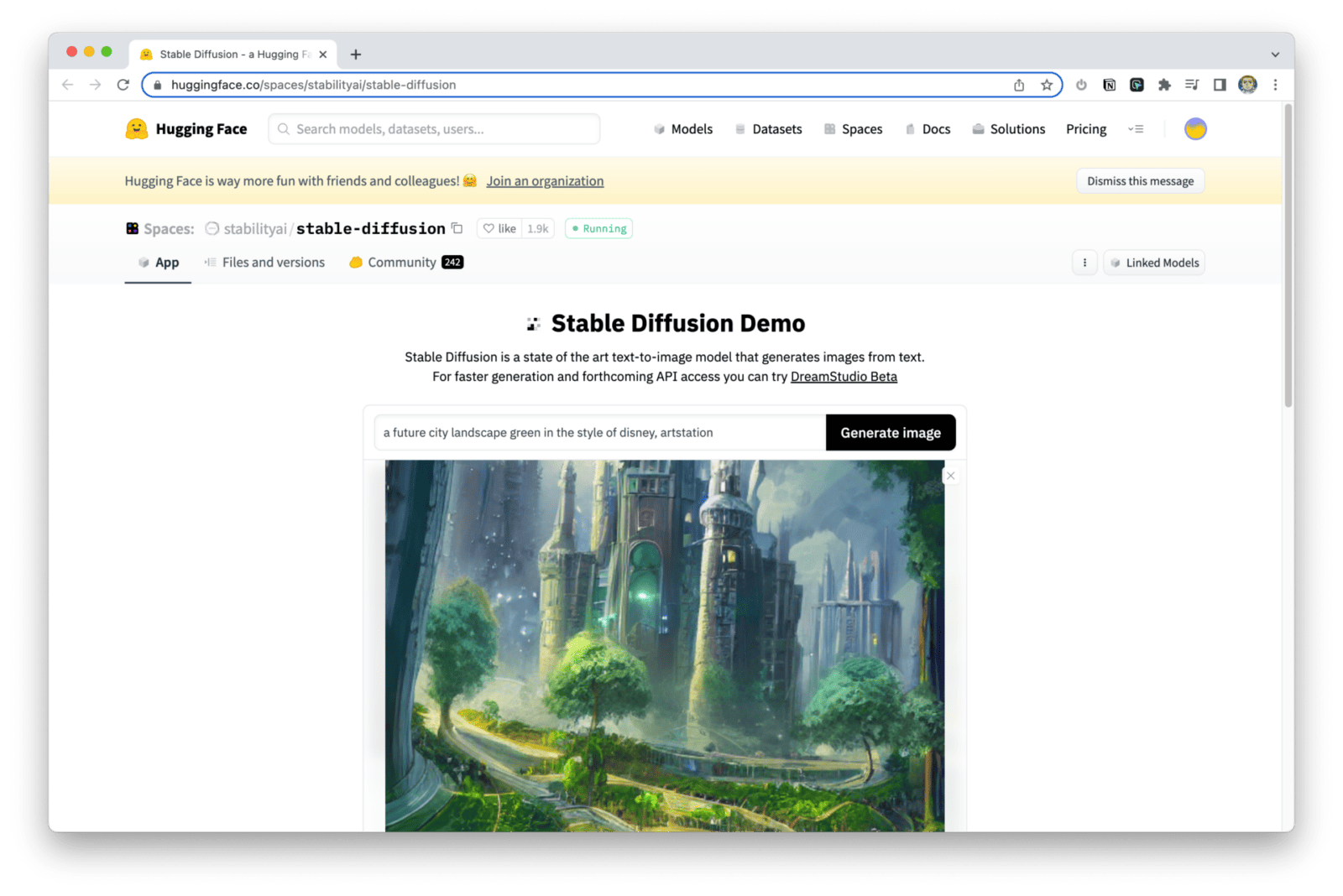
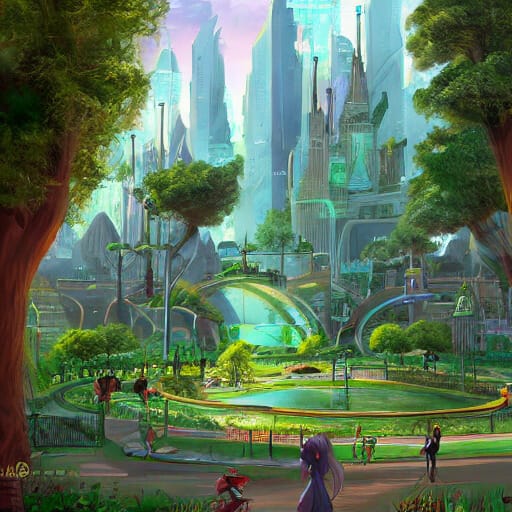
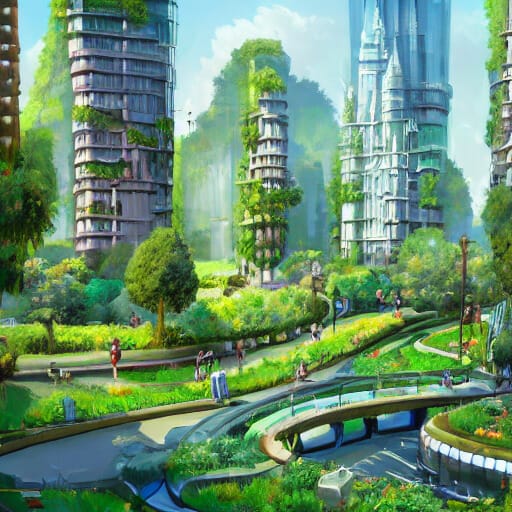
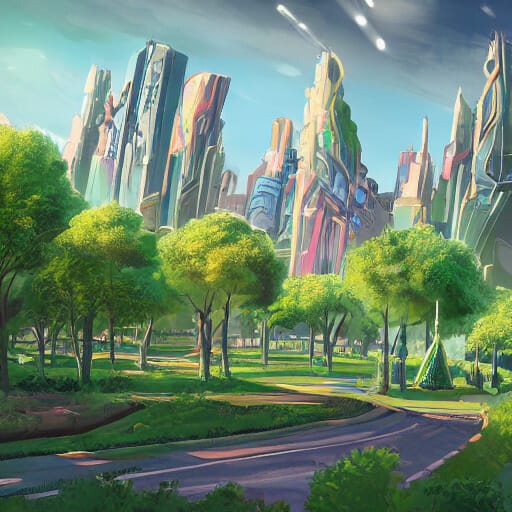
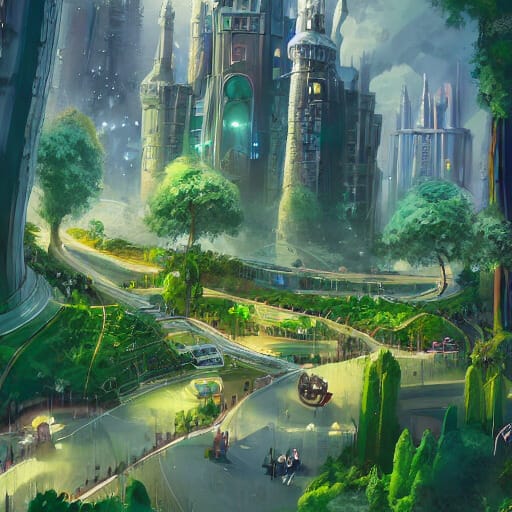
And the fun part: It’s for free! There’s no limitation in terms of credits and it’s a perfect playground to get used to how text-to-image prompts work in general. As mentioned before when explaining midjourney or Dalle-2, I recommend reading through some text prompts… or as the magic of open source already unfolds, use sites like lexica.art and look at the eye candy and copy prompts.
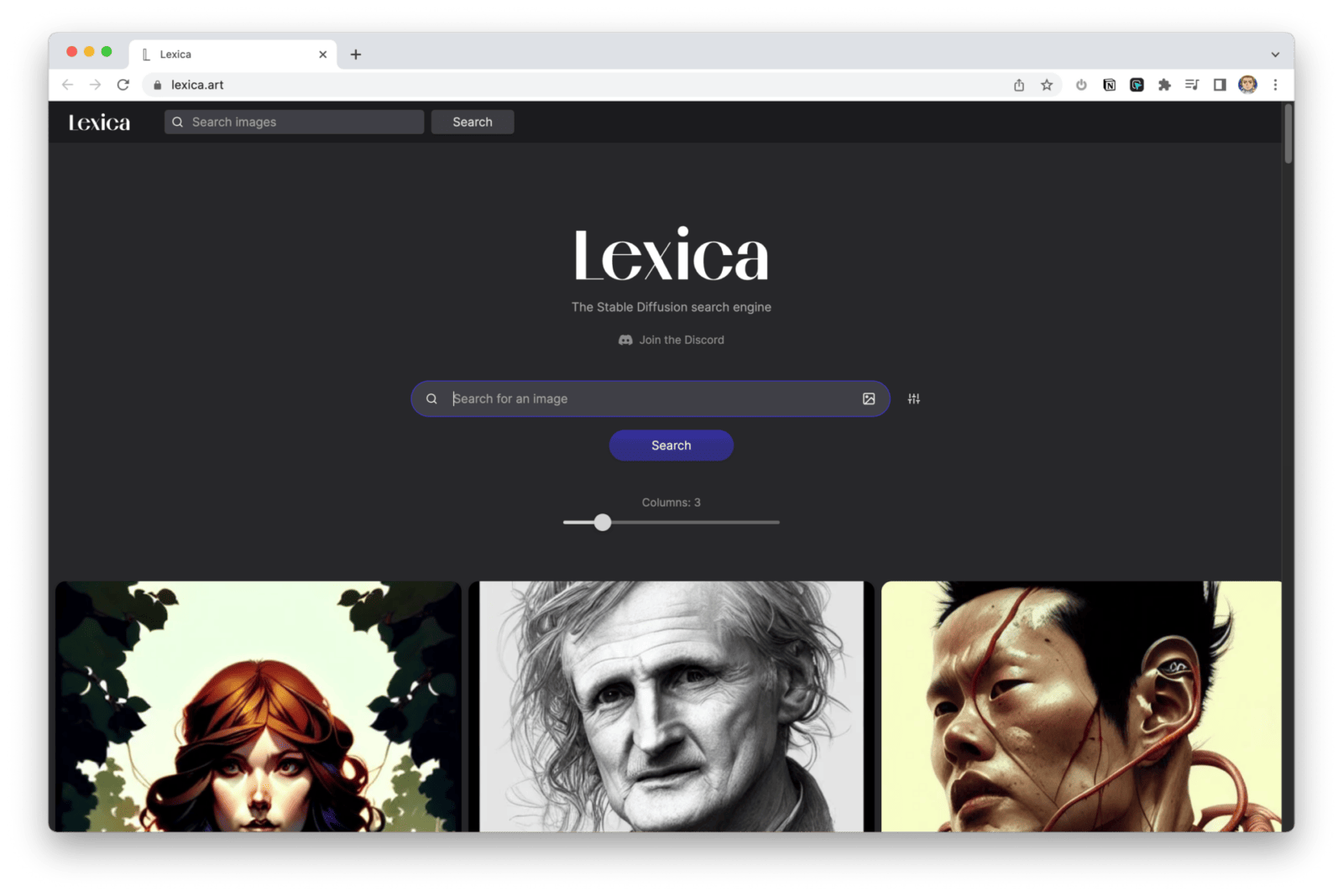
As an alternative to lexica.art, you could also try arthub.ai.
Now I almost forgot to quickly explain what diffuse the rest could do for you… it’s kind of the beautifier of your paintings. Draw like in MS Paint and add a text prompt, about what your line drawing might be. In my case presented with an owl (owl got very popular in all this process of text-to-image generation) – the transformation of kid paintings is also quite fun 😉
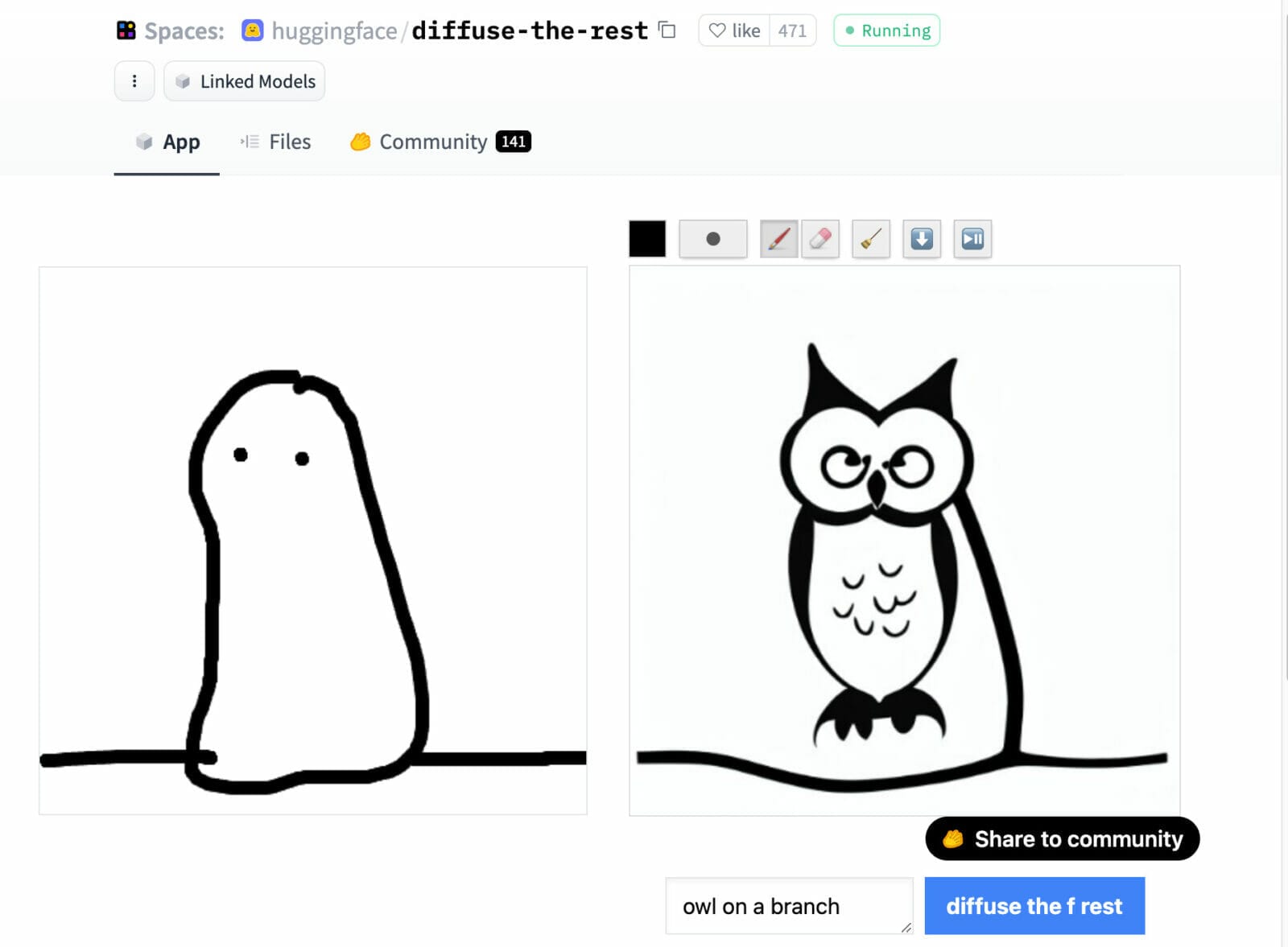
Stable Diffusion on Google Colab
Google Colab is another resource you need to know when it comes to working with Python in the context of machine learning. Google Colab makes data science, deep learning, neural networks, and machine learning accessible to individual researchers who cannot afford expensive computing infrastructure.

Edan Meyer explains how to use google colab with his own modified colab notebook in his great video “Stable Diffusion – What, Why, How?”. He is explaining the whole process to create images, similar images or even videos. A deeper understanding of how using the code works help to unleash the full creative potential.
In very simple words, you open a colab notebook and follow from top to bottom. On the left you find some little play icons to execute. After executing one after another, there might be some text prompts or variable to modify to lead you to your result.
Local Apps and other Web GUIs for Stable Diffusion
Since we have the first open-source release, it feels the market is exploding. I saw downloadable apps to come and go within 2 weeks, so it’s hard to give you a list of all the options there are. Also, there's no guarantee they are still working. Many people are fascinated by these tools. Some want to make money quickly, others want to share, and everyone wants to keep improving the technology.
So, I start with another Web GUI for Stable Diffusion called peacasso:
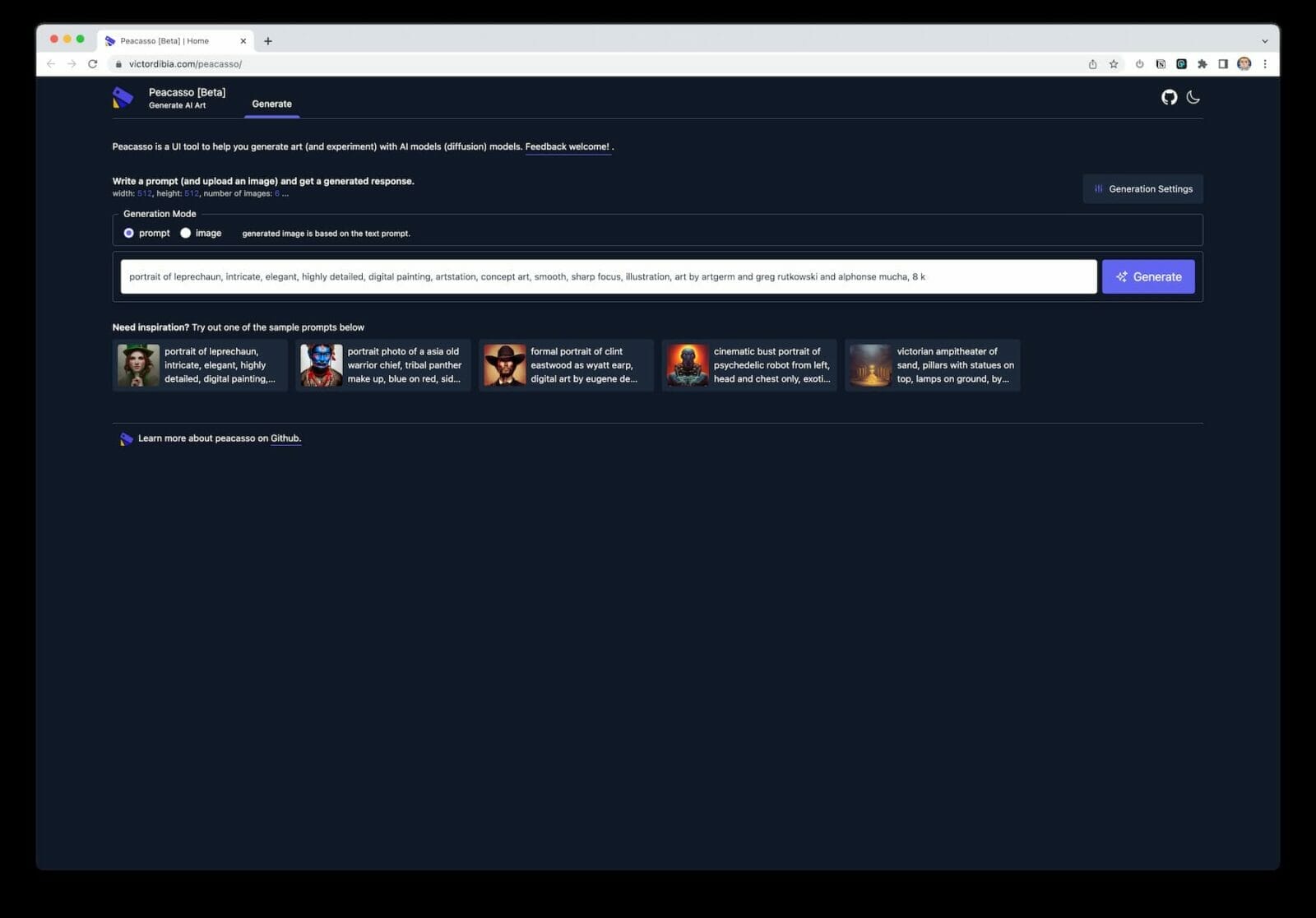
And here’s a desktop app to run Stable Diffusion for the mac (m1,m2) called DiffusionBee:
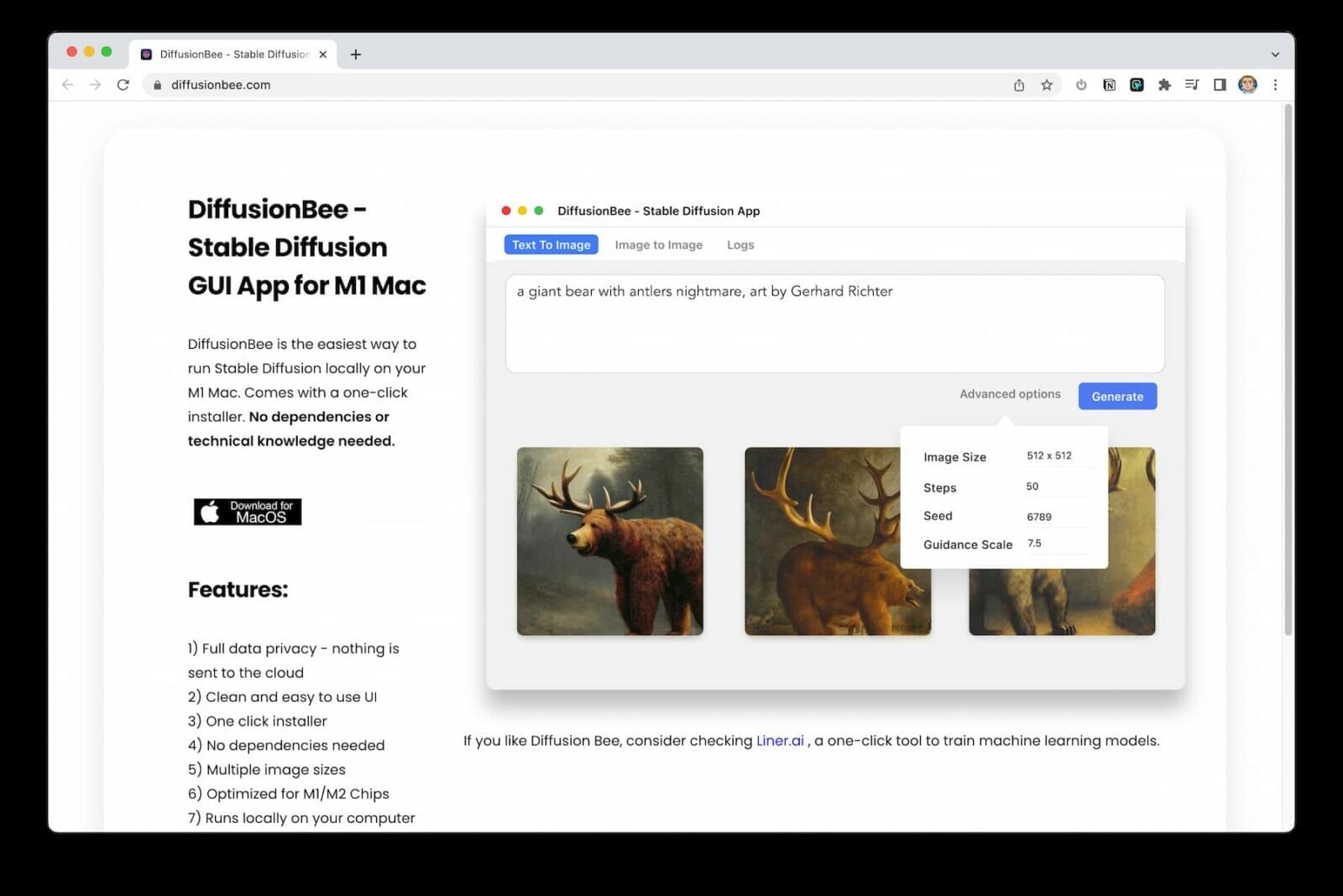
Feel free to share more cool apps, sources in the comments. As of Mid September 2022 there were so many tools, it is hard to keep an overview.
Replicate is another interesting cloud API I wanted to give a try. If you use the tools you will notice, rendering takes time. Using a cloud API for rendering can improve energy efficiency and reduce your carbon footprint, while also decreasing rendering times.
Explore the offerings of recplicate.com, there’s a clean Stable Diffusion install as well as other interesting tools to help you to optimize your images or get a desired effect.
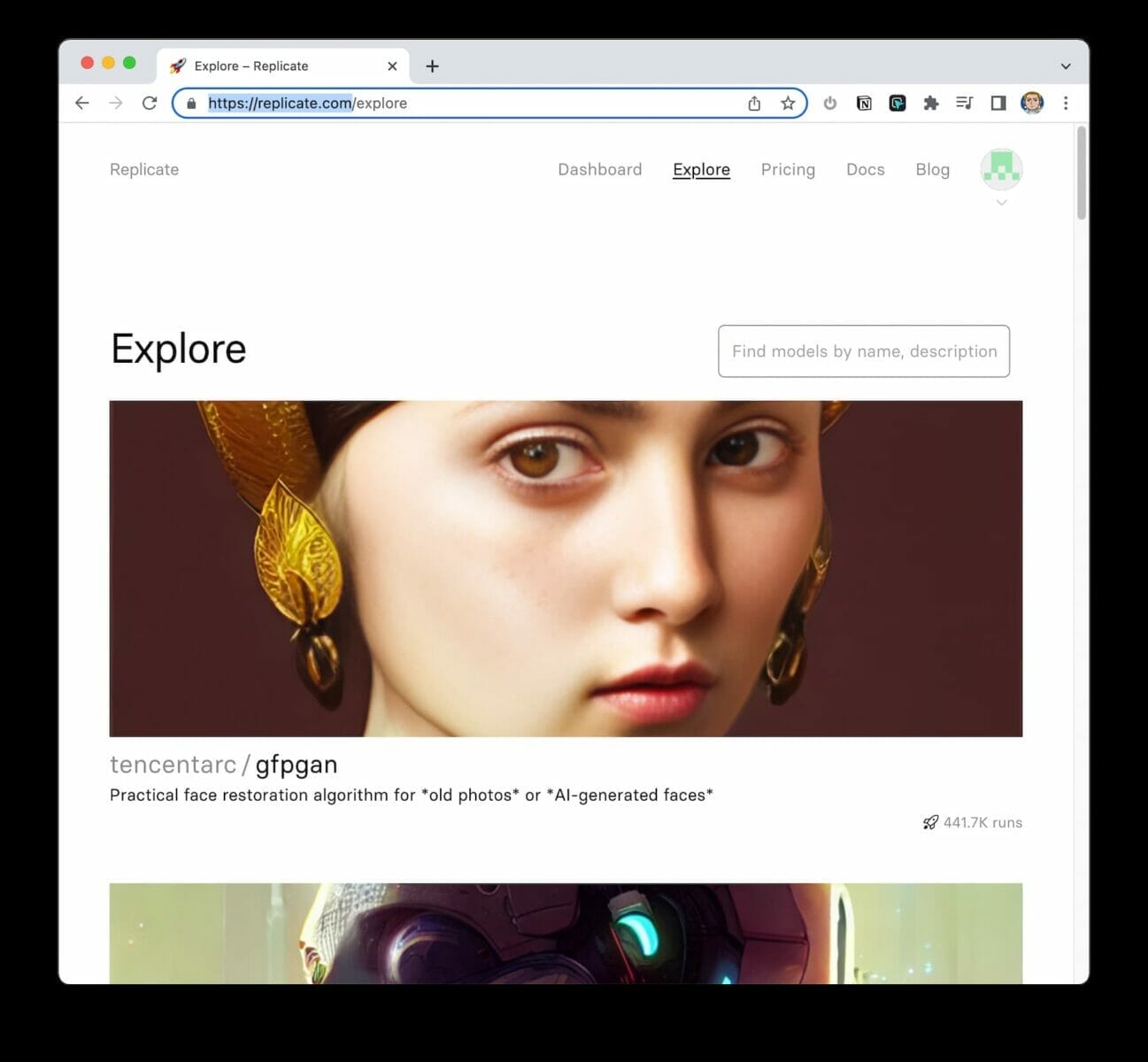
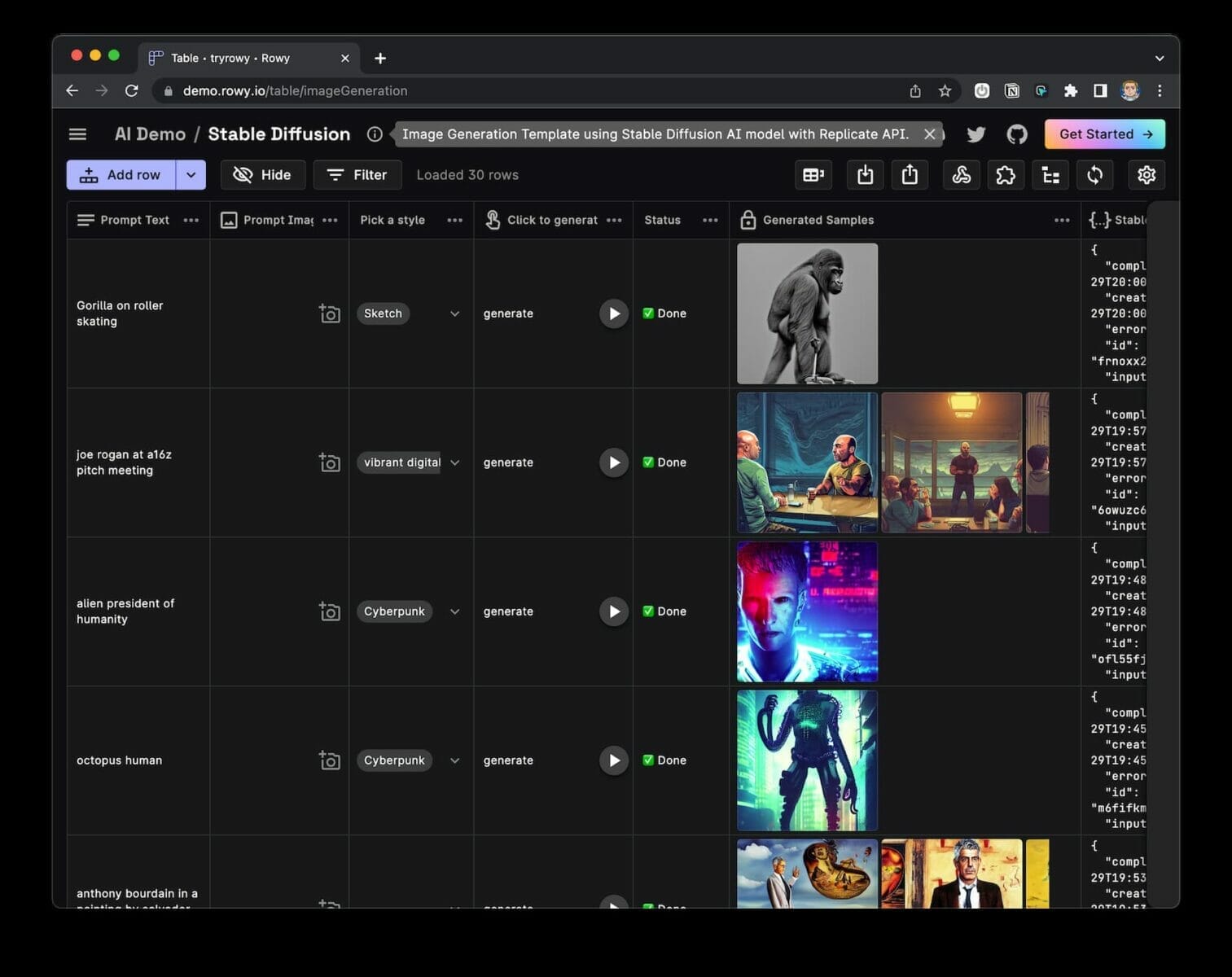
Stable Diffusion – local installation on MAC M1
Finally, some thoughts and links on installing Stable Diffusion locally on your computer. I personally only installed it on an M1 Macbook. With the advantage of the RAM being able to be shared.
There's a tutorial on options to work with Stable Diffusion locally I discovered while finishing the article, but it seems to be the official one (I didn't test it though as I didn't want to break my running version); the tutorial is from AssemblyAI itself: "How to run stable diffusion locally to generate images".
When I tested my local install, I basically followed along with another introduction from replicate.com: "Run Stable Diffusion on your M1 Mac's GPU".
In addition to the instructions, some extra tips, as I also had some trouble while installing. First, check for the correct Python version. You need 3.10, which could be downloaded and installed from python.org.
If you have trouble to switch the python version, there could be many reasons (I couldn't probably name). I used a version control, as options there were ASDF and pyenv. I decided on pyenv and followed the instructions and had no further issues to switch the version.
Another hint I could share with you... watch your folders. Always execute the prompts from the correct folder and be prepared to wait a little for your prompts to be executed.
Here are some renderings on sculptures from my local tests:
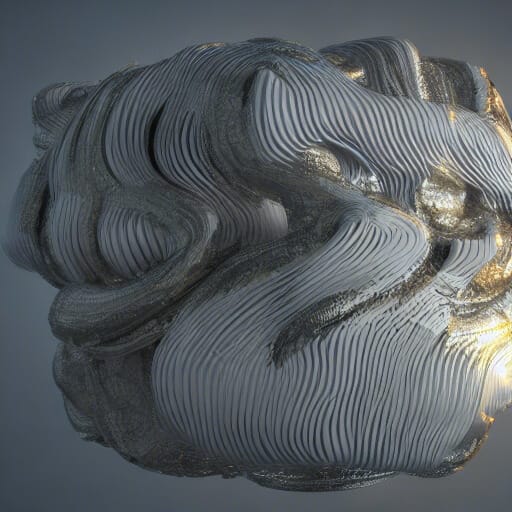
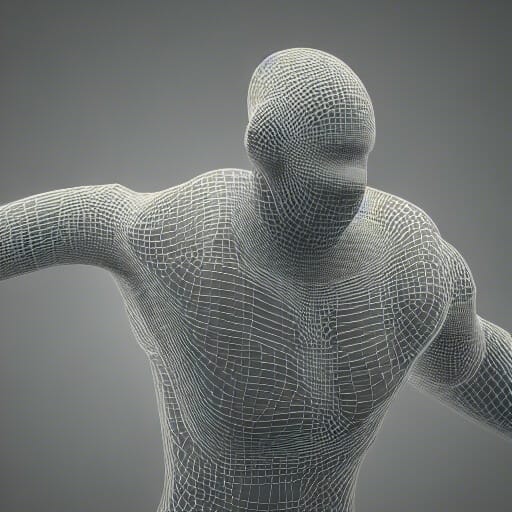
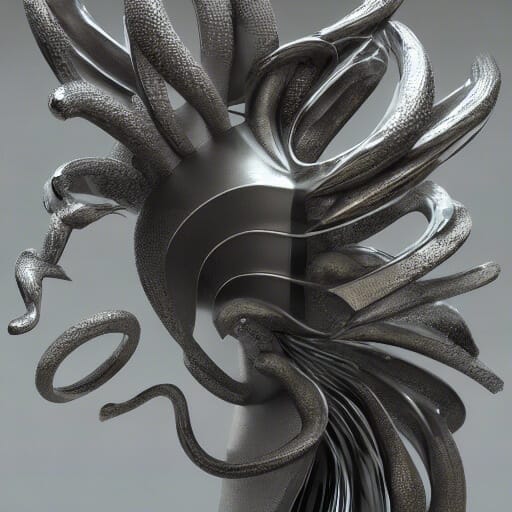
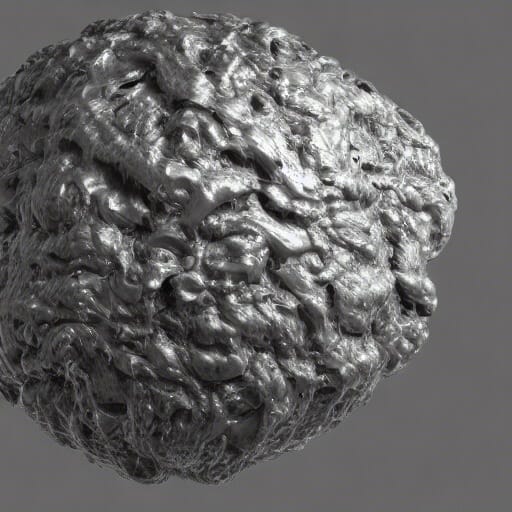
Stable Diffusion – local installation on PC
It took me a little while to test the PC installation, but I guess this guide here is quite good and straightforward:
AUTOMATIC1111's SD-WebUI (Windows)
Following the guide you have similar steps as on mac, but it is simpler in the execution as there are more installers to run. Finally you have a webGUI to get going.
Please note, that you need a GPU with at least 6 GB of VRAM. 10 GB or more on a NVIDIA GPU with CUDA is highly recommended to allow larger image sizes. A business card, such as the RTX A series is preferred as the energy consumption compared to the value is much better. Maybe the upcoming NVIDIA generation will bring even more value.
On the tutorial site you also get some other guides (I didn't test):
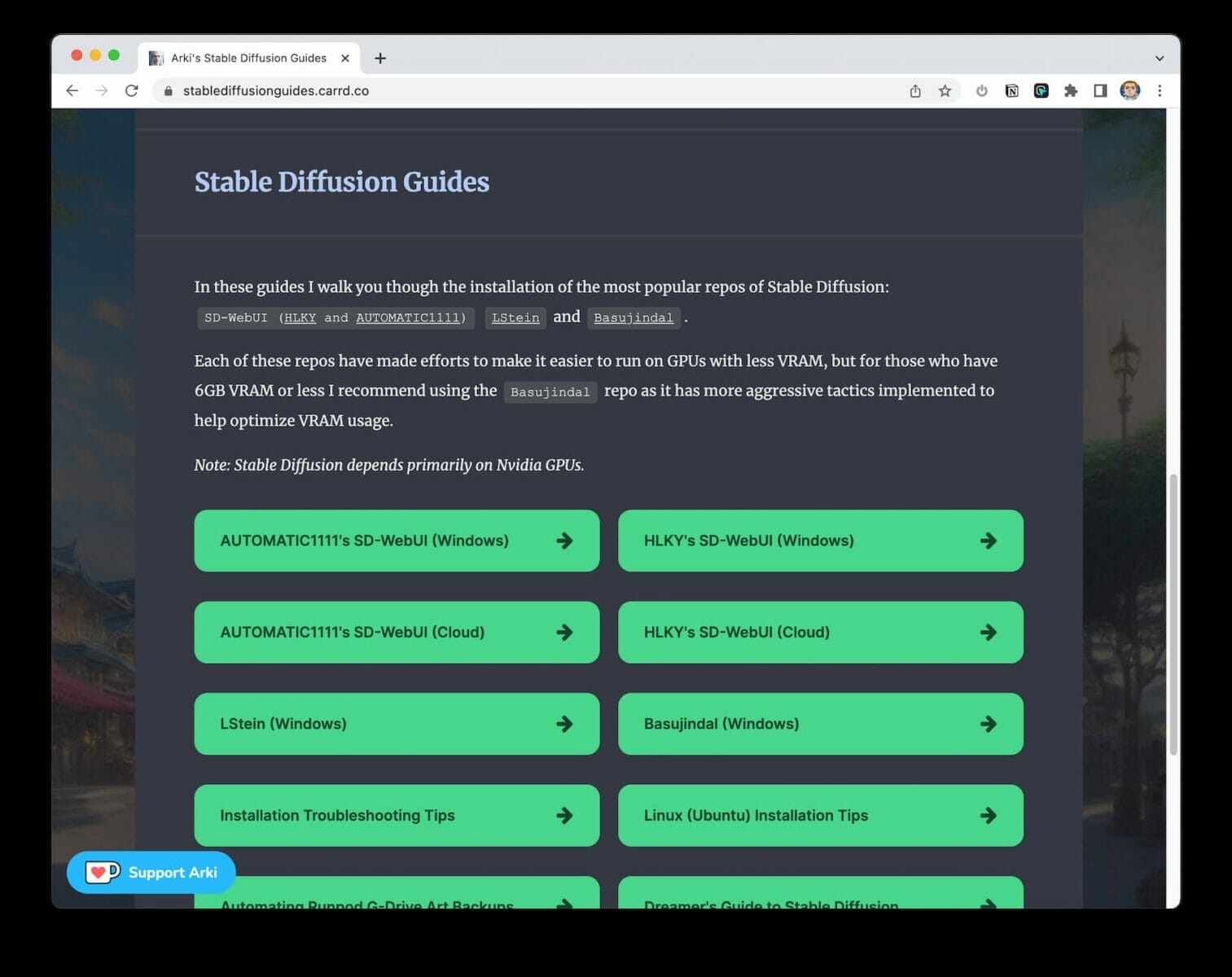
Stable Diffusion – other installs
Creativity unleashed with Stable Diffusion
To get an idea about the full potential I collected some random examples from the past month. There are so many exciting opportunities. People create comics, movies or just play around.
Kali Yuga models
Kali Yuga provides some cool custom-trained models for Stable Diffusion. Here are some shots taken from Twitter:
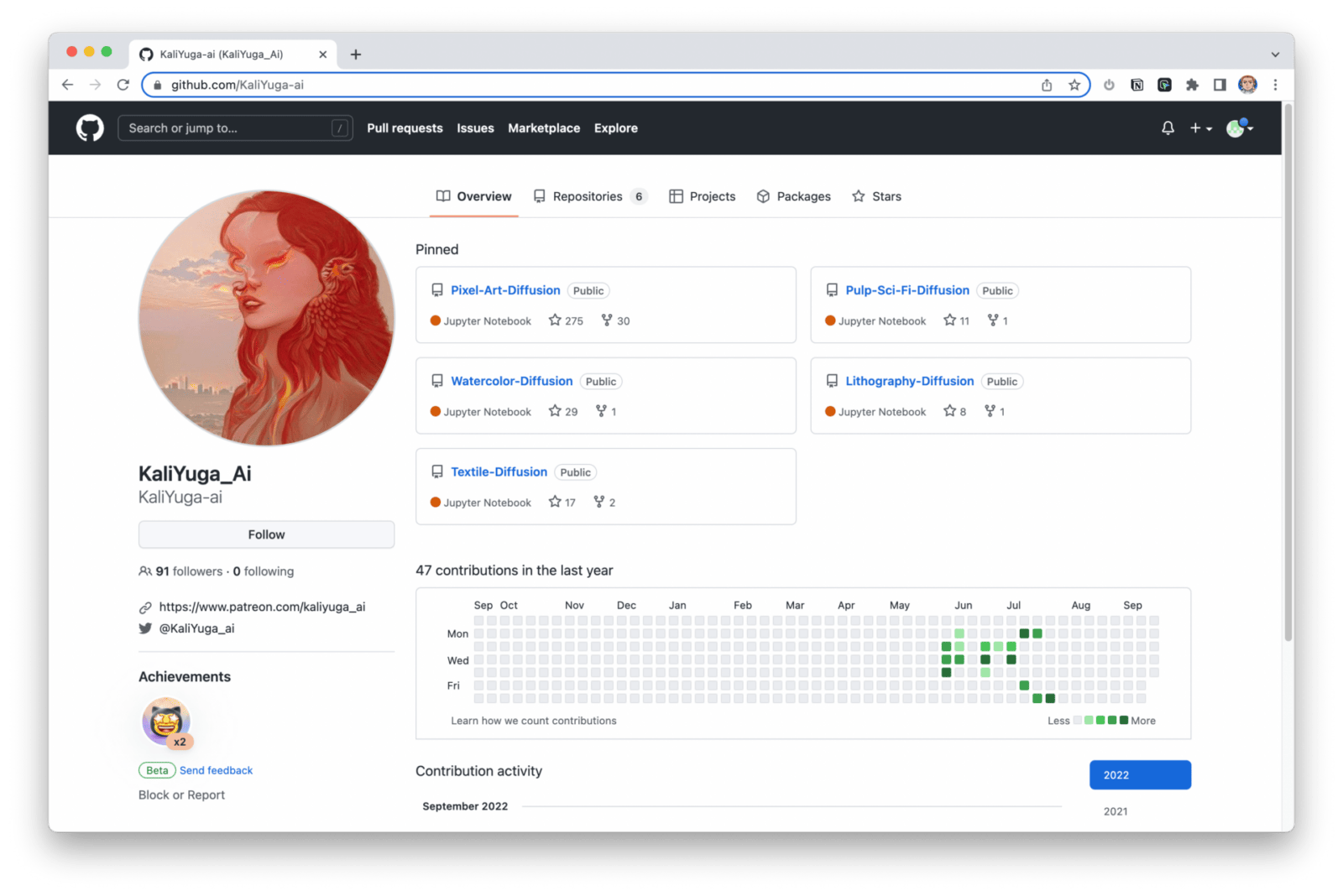
You might have guessed it, there are also notebooks on google colab around to work with them. An alternative would be jupyter notebooks, but to keep it simpler, we stay with google colab.
- Notebook for #textilediffusion.
- Notebook for #Pulp-Sci-Fi-Diffusion
- Notebook for #lithography-diffusion
- Notebook for #watercolor-diffusion
- Notebook for #pixel-art-diffusion
Those notebooks are quite easy to handle. I used the #textilediffusion, to generate this sample piece here:
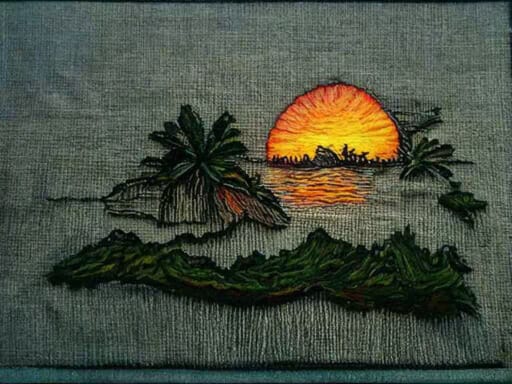
Click the images to jump to the examples. Due to data policy, I don't embed external sources.
Stable Diffusion and Music:
Stable Diffusion and 3D
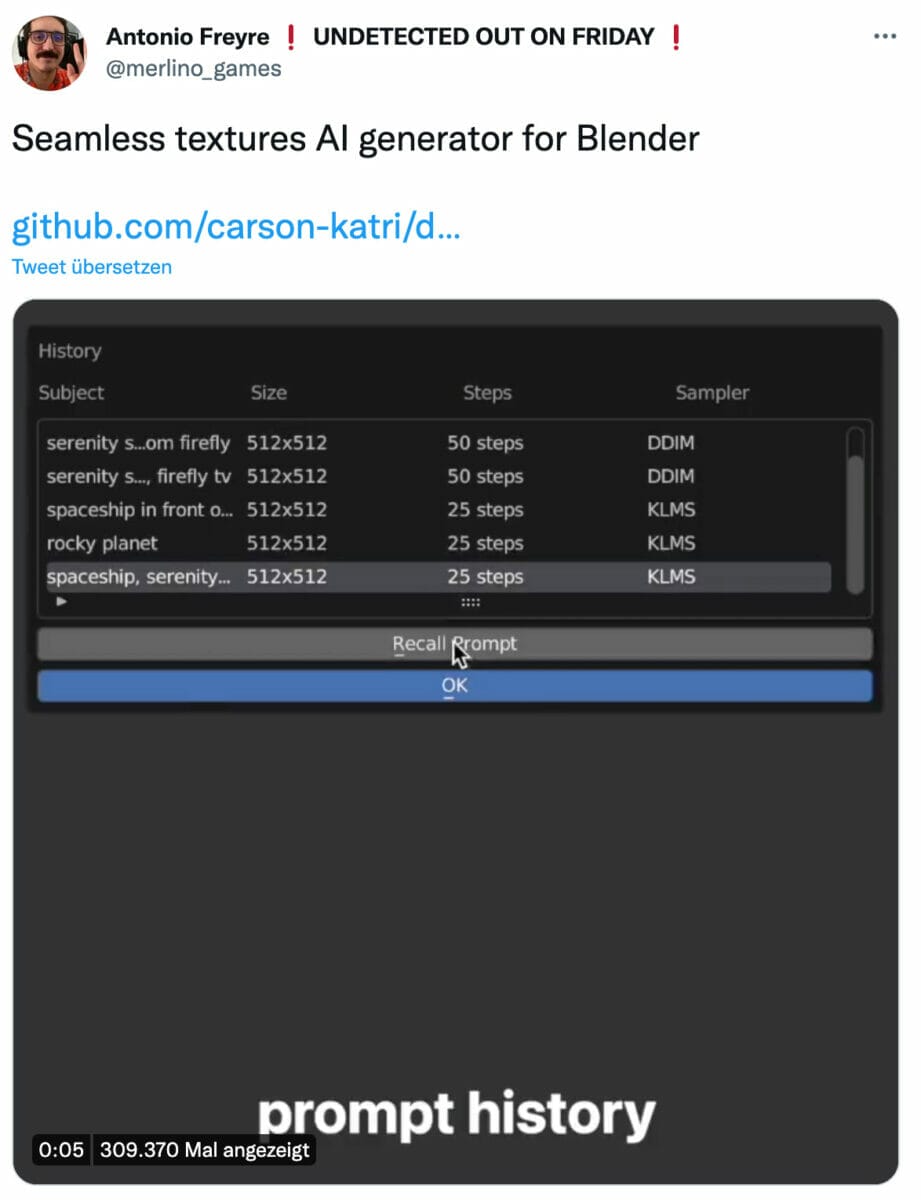
Here's the GitHub Source for the Stable Diffusion blender texture generator.
Another link, that is connected to Unreal and midjourney is the generation of textures.
Stable Diffusion and Image Tools
Stable Diffusion Photoshop Plugin
There are also options for text-to-image in Canva and GIMP already and Nightcafé and artbreeder seem to have changed their models towards stable diffusion as well (you might remember that earlier this year they both used some GAN+CLIP solution, as mentioned in my article).
Stable Diffusion to generate color palettes from text descriptions
Other sources to mix with Stable Diffusion or to watch in general:
- Get3D: A Generative Model of High Quality 3D Textured Shapes Learned from Images
- OpenAI Whisper
- Google DreamBooth: Fine Tuning Text-to-Image Diffusion Models for Subject-Driven Generation (GitHub implementation for Stable Diffusion and Google DreamBooth and another Stable Diffusion Implementation with DreamBooth)
Final thoughts on Stable Diffusion AI – image jockeys on random turntables.
I love it. Going for the open-source path is so great to unleash new instruments of inspiration and with lucky punches, even to create art. Art is expressive and the artworks being created might get expressive. For me it's an enhancement of our daily practices being creative. I also don't think anyone must be afraid of losing jobs. For precise tasks it is still tricky to work with the tools.
The models are only as good as the inputs; handling the inputs is not the easiest practice and as generating the images takes some time… in terms of client work, the way using any generator might not be the fastest option to choose. As always, I assume clients want to negotiate cost as they think everything is going easier now. But currently the image generation at a professional level is still not precise enough. But the tools are already there to help. Google Dreambooth for example could help a lot to bring consistency into image series.
Looking at the development speed I assume the topic about precision will be a matter of the next years, as well as the topic about speed and custom training sets. I am also worried a bit about the footprint being generated from all the image generations (including all the learning upfront). In hugging face there's a note on the Stable Diffusion model card itself: 11250 kg CO2 eq for the learning (calculated with the machine learning emissions calculator).
The development in text-to-image is far from its boarders yet. But it's so impressive to see the speed of the community to adopt these technologies.
Currently the tools help to discover creativity to finally create a mix of outputs we have seen before. In the future I am sure we can use the techniques to do whole mood boards for clients and in some cases even final graphics or artworks for them.
The only art I’ll ever study is stuff that I can steal from.
David Bowie
Like the evolution of web bandwidth, I think we need some more speed in terms of image generation to open new options and create beautiful human-centered-artworks as one day maybe images could be generated in real time based on likings of visitors. I also assume, at the moment we reached a certain grade of automated artworks, more and more people will enjoy discovering real artworks with their hands again and value of the real original artworks will raise.
In general we shouldn't trust media without a critical perspective, especially when we see images. History showed us that people will abuse technology. So I hope for people to find value in real in-person events after Covid pandemic again. Digital could and will be a lot of fake.
The use of some kind of style transfers on Smartphones is very likely by next year. Dynamic Video generation is also on its way (see make-a-video from meta ai), so we have text-to-video generation and music, VR worlds and games are also around the corner, as Midjourney founder David Holz said. Due to open-source development, I won't say it takes 10 years. And also Google Colab and other services to run the software will change their business models to profit from this boom.
Advertising or Branding will also be affected. It will be an exciting journey in the next two or three years. Osmosis pretends to have some of the features that could be interesting for an early stage. Just imagine adding a target group to the prompt, an industry or a segment. For Learning there are many galleries out there, such as dribble, FWA and many more 🙂 it will be fun to see where this journey goes! (Saying this not being in an international network agency anymore).
As mentioned many times, the development speed is incredible. The current hot tool is google dreambooth (connected to Stable diffusion). Every month there might be another unexpected cool tool to pop up. Maybe even photoshop integrated the tools for their next release.
For me, design turned more into managing design over the years but still design is functional, and art is expressive. Currently it feels easier to create automated expressiveness in fantasy worlds, but nonetheless I also use AI tools during the creative process. The results of machine learning give you a condensed view of common thoughts. So, I often used the prompts to check for color ranges of certain clients, compared it, what google says and easily created something one could call the "wisdom of the net" result.
Our traces on the net and our databases of artists created the look we could mix now. We are the image jockeys of generations that are already there. Unfortunately there's still a lot of randomness in the result to utilize it more professionally.
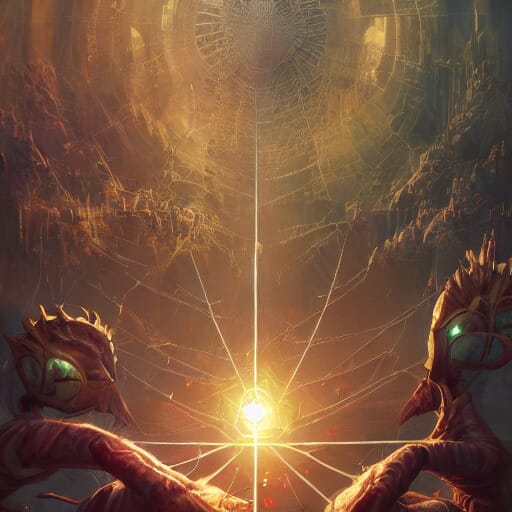
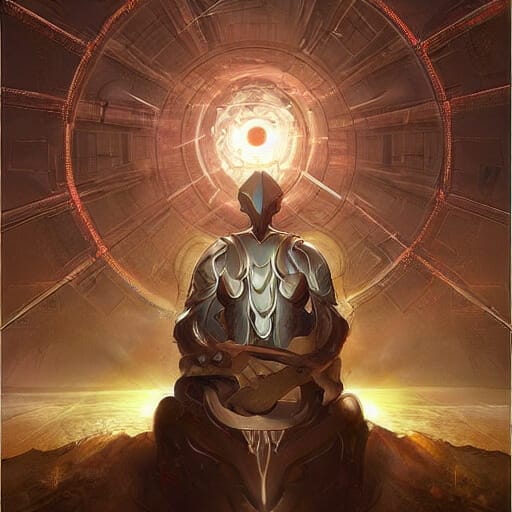
So long, enjoy and go out and play to discover the fun playing with those great tools!
As mentioned, it is nearly impossible to keep up with all interesting developments.
Google now has imagenVideo as a response to Metas Video diffusion solution: https://imagen.research.google/video/
And there are a lot of other interesting developments as well:
- 3D generation from a single image: https://3d-diffusion.github.io/
- Text-to-3D "Stable dreamfusion": https://github.com/ashawkey/stable-dreamfusion
- Human motion diffusion models: https://guytevet.github.io/mdm-page/
This Article was mentioned on manfred-feiger.com